Hentet: https://www.itnonline.com/article/how-invest-success-digital-pathology%E2%80%99s-ai-ecosystem
Study findings prove useful for prospective stakeholders in understanding the influence of adjacent markets of enterprise imaging and laboratory information systems
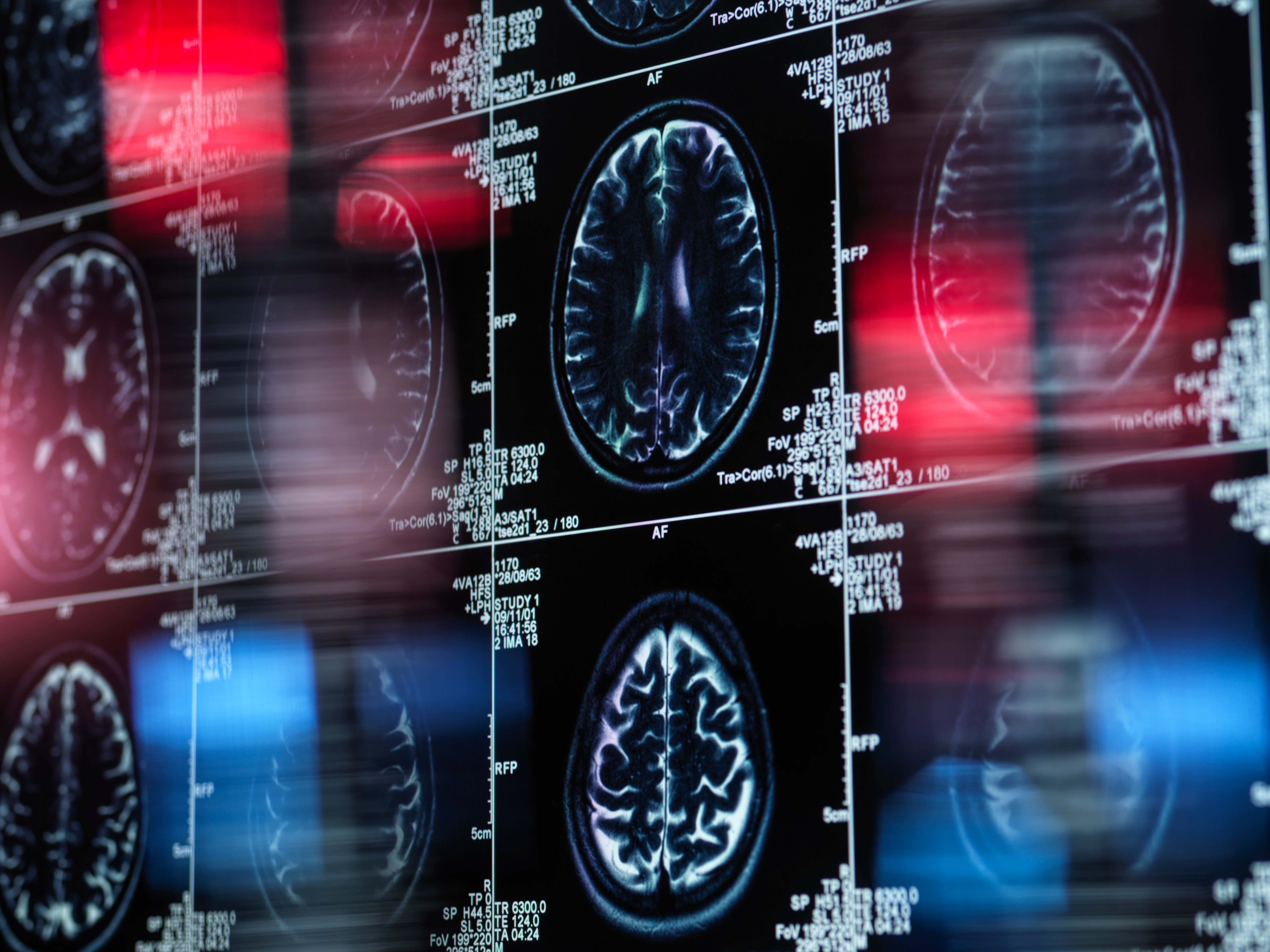
Digital Pathology’s ecosystem will not support its artificial intelligence (AI) vendors indefinitely. So how should investors and partners differentiate between players in today’s market?
There is an abundance of vendors currently developing their own products in the AI ecosystem for digital pathology (DP), and the market is simply not ready to sustain every vendor in operation indefinitely. Consolidation has already begun — note the recent acquisitions of Crosscope and KeenEye, yet new entrants are still expected over the next few years, keeping the market crowded.
Several vendors are also currently seeking investments and partnerships to help develop their businesses further. It can therefore be a challenge for third parties to navigate the AI ecosystem and successfully differentiate between vendors in a meaningful way.
Signify Research recently published its AI in Digital Pathology deep dive, “Digital Pathology Market Intelligence Service – World – 2023,” the second deliverable of its market intelligence service. Following is an excerpt of some of the key findings from this study, which may prove useful for prospective stakeholders looking to invest their time, money and reputation in DP AI.
Ultimately, the question I am looking to answer on behalf of these stakeholders is this: how can stakeholders ensure that they invest in a solution that will be a success?
Understand How the Vendor is Planning to Approach its Ecosystem
Figure 1
The above diagram briefly outlines the many types of vendors currently developing their own DP AI algorithms. Note that many of these have existing partnerships with one another, and some are more proactive than others.
One of the key trends driving the general DP market forward today is interoperability. Labs don’t want to be tied down/restricted to a single or select group of vendors. They want the freedom to be able to select any of the best-of-breed products as and when the requirement arises.
Therefore, each AI developer will need to make an important decision as they commercialize their products:
•Will they integrate with other third-party platforms? And will this strategy be pursued strategically or on an ad hoc basis?
•Or, will the vendor choose to develop its own platform to host in-house and third-party algorithms?
Both approaches have their own strengths and weaknesses, however what is clear is that there is no way forward that relies on one vendor as a silo.
On that note, I would encourage potential investors to examine their prospective investment’s partnership strategies closely, as the key to a successful algorithm is making it as widely available as possible.
Understand Vendor Types and their Respective Strengths and Weaknesses
As can be seen in Figure 1, I’ve divided the ecosystem into roughly eight types of vendor, each with their own strengths, weaknesses, opportunities and threats. The table outlines these briefly and addresses the relative impact each is set to have on the DP AI market over the next few years.
As the table above shows, each type of vendor has unique advantages and potential disadvantages shaped by its background.
While some of the lines between vendor types are blurring as the market evolves (think AI incumbent/image analysis specialists developing image management software), each will shape its strategy based on these strengths.
To thoroughly evaluate a vendor, investors and partners must understand the nuances of each company’s background and be informed how each will take advantage of its strengths and mitigate any disadvantages to win opportunity over similar competitors and other vendor types.
Understand Geographical Nuances
Other factors that may impact a company’s ability to successfully commercialize its product(s) include its location; as alluded to in the last insight, not all geographic markets offer equal opportunities. Some regions are already much more digitized than others, and thus inherently more likely to adopt AI sooner. So, I encourage you to ask:
•Which markets are key to your partner’s early commercialization strategy?
•Is their domestic market one that will embrace home-grown AI expertise?
•Is the vendor situated in a market set to experience stagnated growth in the next five years?
If the last question is true, then a keen strategy to commercialize internationally must be in place, along with evidence that the vendor understands its core targeted markets.
Some customers, such as those in Asia for example, typically prefer bundled purchasing to consolidate spending. Standalone AI vendors therefore may struggle to commercialize in these regions and should have a partnership/development plan in place to tackle this.
After all, it’s not always the quality of a product which dictates a company’s success.
Establish and Verify the Value in the Product
This point is perhaps the most obvious, however, due to the complexity of pathological diagnosis, and the sheer number of algorithms and products in the market today, differentiating between products can be the hardest challenge.
The simplest way to evaluate the commercial prospects of a product is of course to speak with its users. This may not always be possible however.
Here, summarized, are a few of the core product types available today and some of my thoughts on respective potentials.
1. AI for QC. It can be embedded on the scanner or be decision support AI utilized as a second-read quality control (QC) check for diagnosis. While QC for scanners likely has the most potential to be widespread in the market (a significant amount of value can be derived from ensuring slides do not need to be re-scanned), it is also highly likely to become commoditized as a product. Standalone vendors therefore are higher risk, as scanner vendors are also likely to be self-developing these capabilities which will be fine-tuned to their scanners and priced competitively.
AI for a second-read, in contrast, targets a subtle entry to the clinical lab intended to alleviate pathologists’ concerns. By not relying on an algorithm for diagnosis, and instead using it as a double-look, pathologists can avoid having to QC check each other’s work and get to know the capabilities of AI in a more controlled way. It is imperative therefore that these solutions disrupt the pathologist’s workflow as little as possible until an alert is made.
2. AI for clinical diagnosis. There are many different types of solutions available in today’s market focusing on hematoxylin and eosin (H&E), immunohistochemistry (IHC) and other types of stained slides. Breast and prostate cancer are by far the most common indications, and it will be integral to understand exactly how a vendor’s software differs from its competitors. Why have they chosen to develop these despite the competition? What comes next and why? Is the development process ad hoc or planned with partners?
Above all, AI must supplement the pathologists’ workflow. If a pathologist can take one look at a slide and derive the same information an AI product is showcasing, what value does it really add? Solutions must be comprehensive and tackle enough of the workflow to make a difference to an individual’s everyday workload. Breadth
of software, and choice, is also inherently tied to this, but is something that will come with time.
3. AI for biomarker development/research. Many AI vendors are engaging in projects with pharmaceutical companies to develop novel biomarkers for personalized medicine. These projects often involve some sort of capital expenditure (CapEx) fee to support the business until a compliance data exchange (CDx) is developed. Development can take a long time but the business, if it performs well, will be supported by revenue from its partnerships. Lucrative royalties/volume-based pricing will then appear once a sufficient product has been developed. It’s likely that these types of vendors will see the most growth over the next five years as life science companies scale up their digital pathology initiatives.
Each type of products listed above possesses many sub-types, more than can be covered in a single insight. However, in summary, I’d advise prospective stakeholders to consider carefully whether a vendor’s unique selling point lies in their product — how is the AI different to what’s already offered? And does this difference matter to pathologists?
One of the golden tickets in the industry will lie in validation studies centered on diagnostic and academic value. Some like Ibex Medical Analytics and Paige are already participating in large studies, while others are years away from being able to say they have proof.
You can wait for vendors to reach this stage of maturity, risk less and earn less, or choose to enter the market at an earlier stage and potentially earn more over a longer period.
In summary, the digital pathology artificial intelligence (AI) ecosystem is incredibly complex, much like its discipline, and for an outsider to truly be able to invest wisely in the market, there must be considerable time spent understanding the ecosystem in sufficient detail.
Imogen Fitt joined Signify in 2018 as part of the healthcare IT team. Since joining the team, Fitt has studied the breast imaging hardware and software markets, covering mammography, ABUS and conventional breast ultrasound on a global scale, in addition to emerging technologies.
Related Digital Pathology Content:
Will 2023 be the Year of Digital Pathology for US Labs?
Growth Projected for Digital Pathology Market Despite Challenges
The Collaborative and Diagnostic Benefits Digital Pathology is Delivering to Radiology
Twelve Predictions for ECR2022